DAVE LAMMERS, contributing editor
Judging by the presentations at the 2018 Symposium on VLSI Technology, held in Honolulu this summer, the semiconductor industry has a challenge ahead of it: how to develop the special low-power hardware needed to support artificial intelligence-enabled networks.
To meet society’s needs for low-power-consumption machine learning (ML), “we do need to turn our attention to this new type of computing,” said Naveen Verma, an associate professor of electrical engineering at Princeton University.”
While introducing intelligence into engineering systems has been what the semiconductor industry has been all about, Verma said machine learning represents a “quite distinct” inflection point. Accustomed as it is to fast-growing applications, machine learning is on a growth trajectory that Verma said is “unprecedented in our own industry” as ML algorithms have started to outperform human capabilities in a wide variety of fields.
Faster GPUs driven by Moore’s Law, and combining chips in packages by means of heterogenous computing, “won’t be enough as we proceed into the future. I would suggest we need to do something more, get engaged more deeply, affecting things done at all levels.”
Naresh Shanbhag, a professor at the University of Illinois at Urbana-Champaign, sounded a similar appeal at the VLSI symposium’s day-long workshop on machine learning. The semiconductor industry has taken a “back seat to the systems and algorithm researchers who are driving the AI revolution today,” he said.
Addressing several hundred device and circuit researchers, Shanbhag said their contributions to the AI revolution have been hampered by a self-limiting mindset, based on “our traditional role as component providers.”
Until last year, Shanbhag served as the director of a multi-university research effort, Systems on Nanoscale Information fabriCs (SONIC, www.sonic-center.org), which pursued new forms of low-power compute networks, including work on fault-tolerant computing. At the VLSI symposium he spoke on Deep In-Memory Architectures and other non-traditional approaches.
“Traditional solutions are running out of steam,” he said, noting the slowdown in scaling and the the “memory wall” in traditional von Neumann architectures that contributes to high power consumption. “We need to develop a systems-to-devices perspective in order to be a player in the world of AI,” he said.
Boris Murmann, an associate professor in the Department of Electrical Engineering at Stanford University, described a low-power approach based on mixed signal-based processing, which can be tightly coupled to the sensor front-end of small-form-factor applications, such as an IoT edge camera or microphone.
“What if energy is the prime currency, how far can I push down the power consumption?” Murmann asked. By coupling analog-based computing to small-scale software macros, an edge camera could be awakened by a face-recognition algorithm. The “wake-up triggers” could alert more-powerful companion algorithms, in some cases sending data to the cloud.
Showing a test chip of mixed-signal processing circuits, Murmann said the Stanford effort “brings us a little bit closer to the physical medium here. We want to exploit mixed-signal techniques to reduce the data volume, keeping it close to its source.” In addition, mixed-signal computing could help lower energy consumption in wearables, or in convolutional neural networks (CNNs) in edge IoT devices.
In a remark that coincided with others’ views, Murmann said “falling back to non-deep learning techniques can be advantageous for basic classification tasks,” such as wake-up-type alerts. “There exist many examples of the benefits of analog processing in non-deep learning algorithms,” he said.
That theme – when deep learning competes with less power-hungry techniques – was taken up by Vivienne Sze, an associate professor at the Massachusetts Institute of Technology. By good fortune, Sze recently had two graduate students who designed similar facial recognition chips, one based on the Histograms of Oriented Gradients (HOG) method of feature recognition, and the other using the MIT-developed Eyeriss accelerator for CNNs (eyeriss.mit.edu). Both chips were implemented in the same foundry technology, with similar logic and memory densities, and put to work on facial recognition (FIGURE 1).
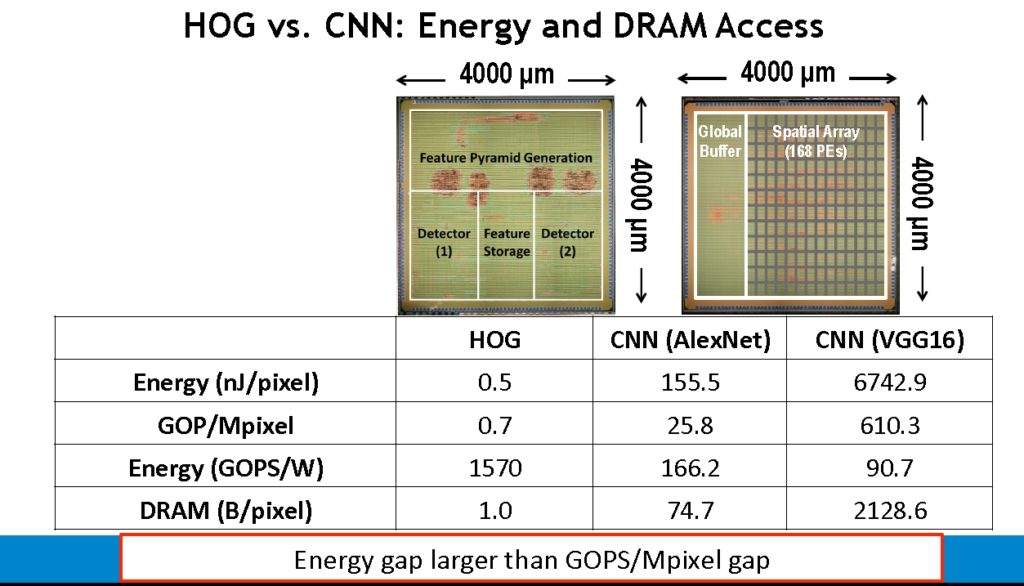
FIGURE 1. Two image processing chips created at M.I.T. resulted in sharply different energy consumption levels. Machine learning approaches, such as CNNs, are flexible, but often not as efficient as more hard-wired solutions. (Source: 2018 VLSI Symposium).
Calling it a “good controlled experiment,” Sze described the energy consumption versus accuracy measurements, concluding that the Eyeriss machine-learning chip was twice as accurate on the AlexNet benchmark. However, that doubling in accuracy came at the price of a 300-times multiplier in energy, increasing to a 10,000-times energy penalty in some cases, as measured in nanojoules per pixel.
“The energy gap was much larger than the throughput gap,” Sze said. The Eyeriss CNNs require more energy because of the programmability factor, with weights of eight bits per pixel. “The question becomes are you willing to give up a 300x increase in energy, or even 10,000x, to get a 2X increase in accuracy? Are you willing to sacrifice that much battery life?”
“The main point — and it is really important — is that CNNs are not always the best solution. Some hand-crafted features perform better,” Sze said.
Two European consortia, CEA-Leti and Imec, were well represented at the VLSI symposium.
Denis Dutoit, a researcher at France’s CEA Tech center, described a deep learning core, PNeuro, designed for neural network processing chains.
The solution supports traditional image processing chains, such as filtering, without external image processing. The modular SIMD architecture can be sized to fit the best area/performance per application.
Dutoit said the energy consumption was much less than that of traditional cores from ARM and Nvidia on a benchmark application, recognizing faces from a database of 18,000 images at a recognition rate of 97 percent.
GPUs vs custom accelerators
The sharp uptake of AI in image and voice recognition, navigation systems, and digital assistants has come in part because the training cycles could be completed efficiently on massively parallel architectures, i.e., GPUs, said Bill Dally, chief scientist at Nvidia Inc. Alternatives to GPUs and CPUs are being developed that are faster, but less flexible. Dally conceded that creating a task-specific processor might result in a 20 percent performance gain, compared with a GPU or Transaction Processing Unit (TPU). However, “you would lose flexibility if the algorithm changes. It’s a continuum: (with GPUs) you give up a little efficiency while maximizing flexibility,” Dally said, predicting that “AI will dominate loads going forward.”
Joe Macri, a vice president at AMD, said that modern processors have high-speed interfaces with “lots of coherancy,” allowing dedicated processors and CPUs/GPUs to used shared memory. “It is not a question of an accelerator or a CPU. It’s both.”
Whether it is reconfigurable architectures, hard-wired circuits, and others, participants at the VLSI symposium agreed that AI is set to change lives around the globe. Macri pointed out that only 20 years ago, few people carried phones. Now, no one would even think of going out with their smart phone – it has become more important than carrying a billfold or purse, he noted. Twenty years from now, machine learning will be embedded into phones, homes, and factories, changing lives in ways few of us can foresee.